Dr. Dániel András Drexler
Associate Professor (John von Neumann Faculty of Informatics)
Research Group Leader (PhysCon, SZTK)
Research topics:
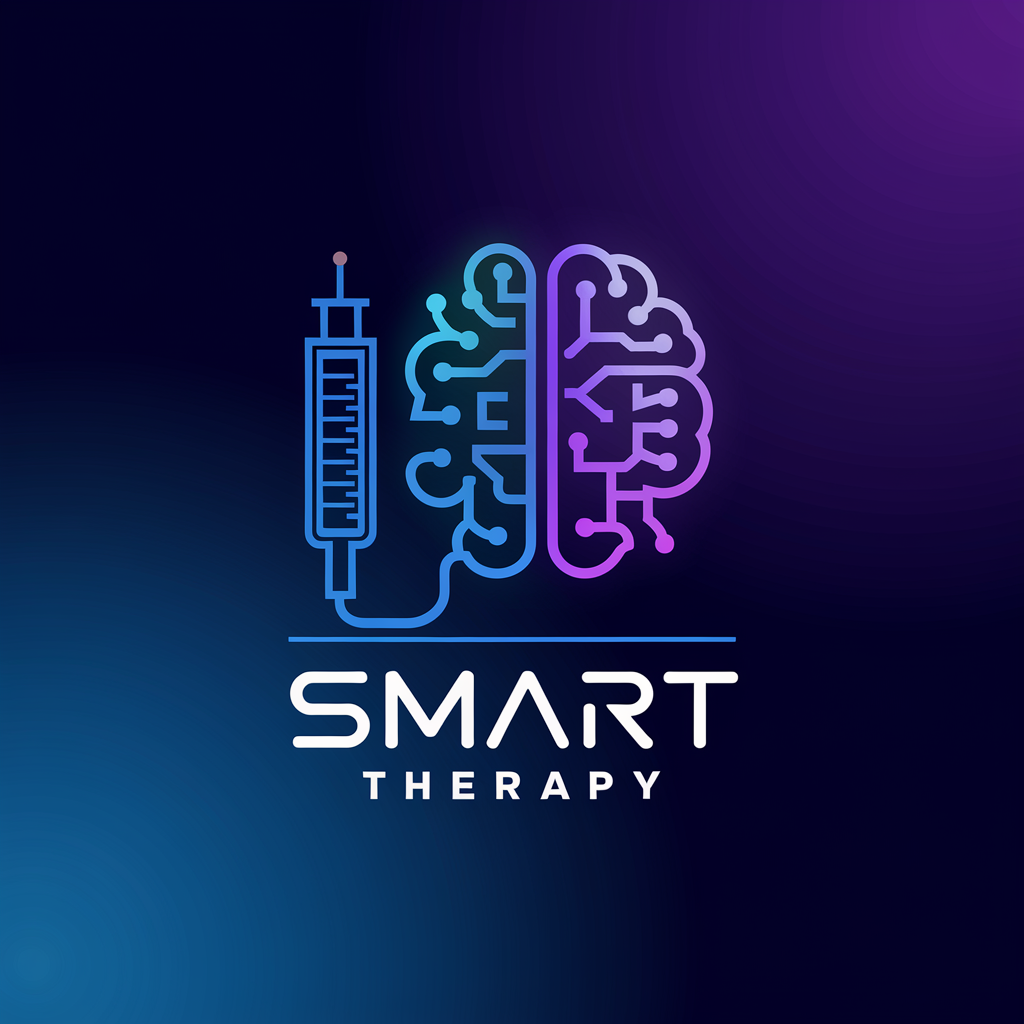
The Computational Therapy Optimisation Research Group aims to develop mathematical and engineering methods to help design personalised treatments by modelling physiological processes and optimising medical therapies. Our main research areas are mathematical modelling of cancer and optimisation of chemotherapy treatments. We aim to develop algorithms that calculate optimal drug dosages based on individual patient data, reducing side effects and maximising survival.
Our research is based on differential equations describing physiological processes, which we personalize through measurements and develop optimisation algorithms to increase therapeutic efficacy. Our model uses a system of four state-variable that takes into account tumor growth, dead tumor cell dynamics, and drug pharmacokinetics and pharmacodynamics. The optimization approach aims to maintain drug levels above a given efficacy threshold with the minimum required doses.
We have developed a range of strategies to optimise drug dosing:
- Personalised therapy for maximum effectiveness – the aim is to keep drug concentrations above the therapeutic limit.
- Minimal dose efficacy – developing algorithms that inhibit tumor volume growth with minimal drug dose.
- Robust therapy – when patient-specific parameters are unknown, a strategy optimised for a wider patient population is used.
Our methods can be applied not only in cancer therapy, but can also be extended to other drug dosing problems, such as optimising antibiotic treatments or other pharmacological interventions. Our research is validated by experimental data and our algorithms are tested on real biological systems to make them applicable in clinical practice as soon as possible.
Major results:
In a paper published in the IEEE Transactions on Systems, Man, and Cybernetics, we present our algorithms for optimizing chemotherapy drug delivery using impulsive systems theory.
Main results:
- We have developed mathematical model-based therapy optimisation algorithms that enable personalised drug dosing, reducing unnecessary doses and side effects.
- Our algorithms have been validated in animal experiments. In in vivo experiments on mice, the methods developed significantly increased the survival time of mice compared to a commonly used chemotherapy protocol.
- We have developed an interval algebra approach that allows the therapy to remain effective even when the uncertainty in patient-specific parameters is large.
- The impulsive control theories used can take into account the periodic dosing of drugs, ensuring effective and sustainable therapeutic strategies.
These results open up new avenues for mathematically based approaches to cancer therapies and may contribute to the development of personalised medicine and future clinical applications.
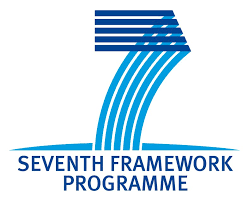
Grants:
2015–2016: FP7-PEOPLE-2012-IRSES-316338 “Dynamical Systems and Applications”
2016–2021: European Research Council Starting Grant ERC-StG 67968 “Tamed Cancer”
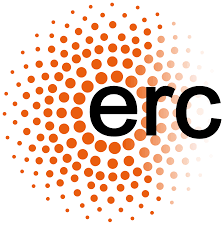
TKP2021-NKTA-36 – Development and evaluation of innovative and digital health technologies
HORIZON-MSCA-2023-SE-01-01-101183111 – DSYREKI – Dynamical Systems and Reaction Kinetics Networks
2020-2.1.1-ED-2024-00346 – Dynamical Systems and Reaction Kinetics Networks
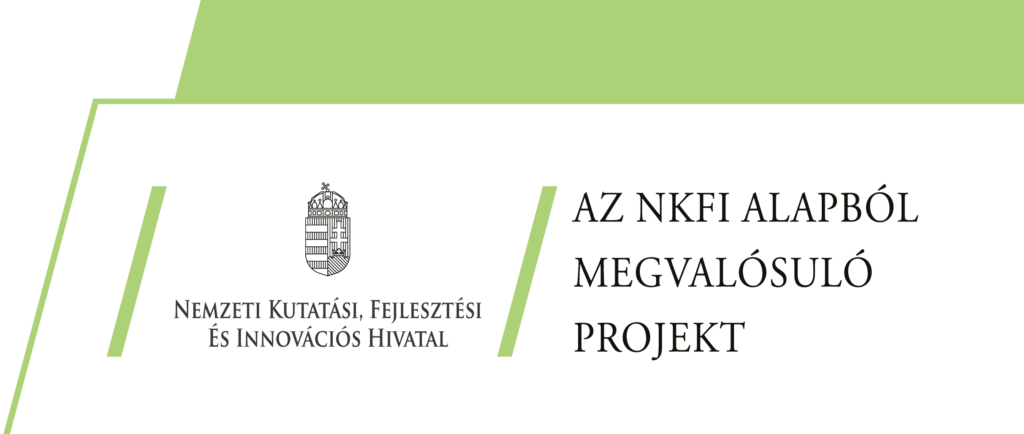
