Dr. György Eigner
Associate Professor (John von Neumann Faculty of Informatics)
Research Group Leader (PhysCon, MHK)
Research topics:
Research topic of the group the Diabetes Research Group focuses on the research, development, and application of engineering methodologies aimed at improving the quality of life for diabetic patients.
Currently, diabetes management is undergoing significant changes due to the increased amount of data from continuous glucose monitoring sensors and portable devices, as well as new therapeutic approaches. Our goal is to leverage our expertise in healthcare data processing and analysis, the development of advanced decision-support systems, and therapeutic algorithms. Our mission includes the development of open-source software, such as metabolic simulators incorporating modern therapeutic procedures, hardware-in-the-loop testing environments for real-time testing of insulin pumps on virtual patients, and the design and execution of human clinical trials. Our active research areas involve applying artificial intelligence for blood glucose prediction, developing healthcare chatbots, and automatic detection of food intake and physical activity. In collaboration with our partners, we conduct clinical data collection studies to understand, mathematically model, or manage through machine learning the effects of everyday events (diet, physical activity, stress) on blood glucose levels.
The Diabetes Research Group is committed to scaling and transferring its knowledge and expertise to other cyber-medical or engineering fields.
The Diabetes Research Group is an active contributor to the Hungarian Artificial Pancreas Working Group of the Hungarian Diabetes Association.
Active projects:
- Algorithm development for automatic insulin delivery
- Model predictive controller development
- Reinforcement learning-based controller development
- Metabolic simulator development
- Research on digital patient representations (digital twins)
- Hardware-in-the-loop testing environment development
- Development of AI-based blood glucose prediction methods
- Clinical data collection study on the relationship between type 1 diabetes and physical activity.
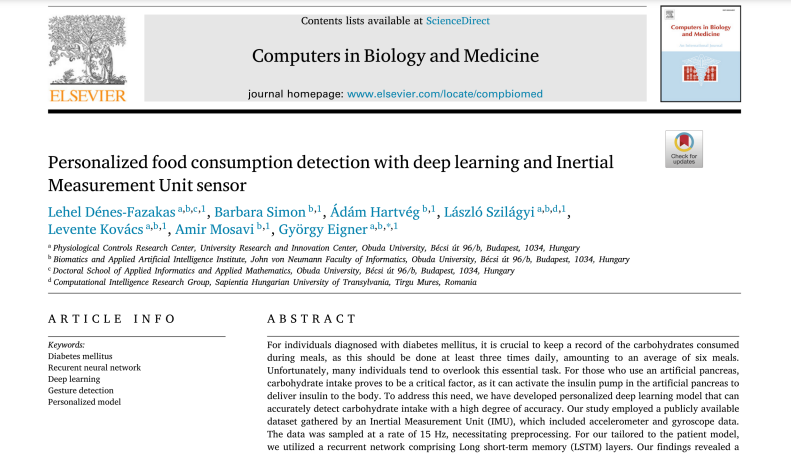
Major results:
• 2023-2025 Clinical data collection study execution
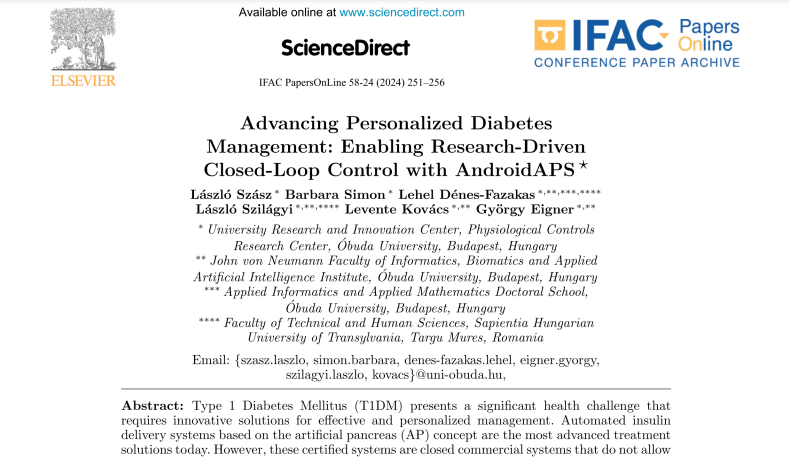
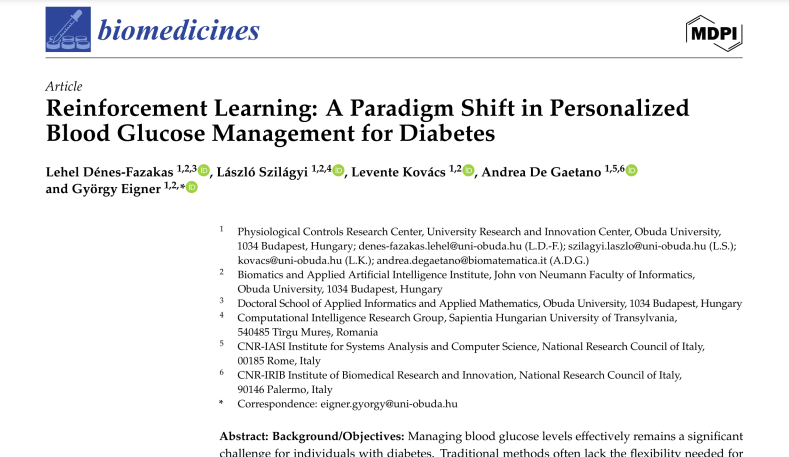
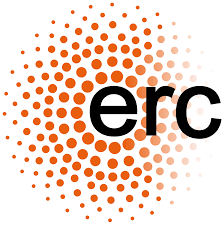
Grants:
2016 – 2021: European Research Council Starting Grant ERC-StG 67968 “Tamed Cancer”
2019 – 2025: 2019-1-3-1-KK-2019-00007 – Establishment of an innovation service platform for the development of cyber-medical systems for diagnostic, therapeutic, and research purposes
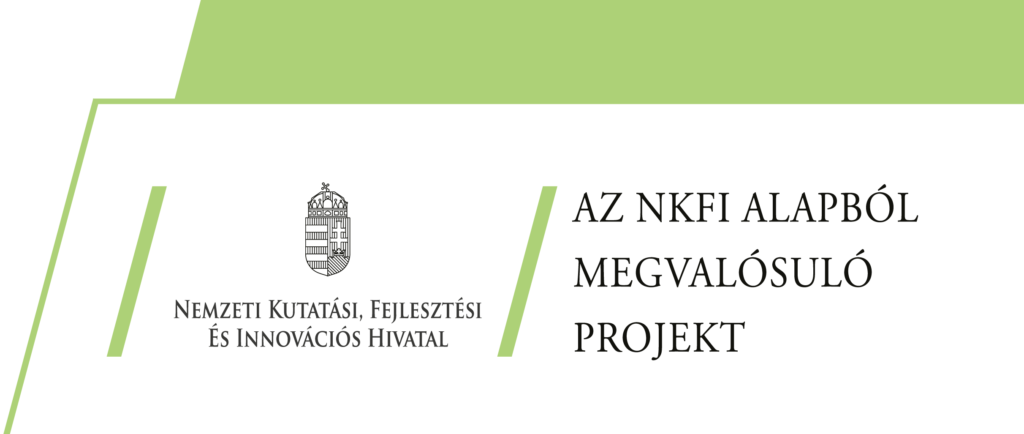