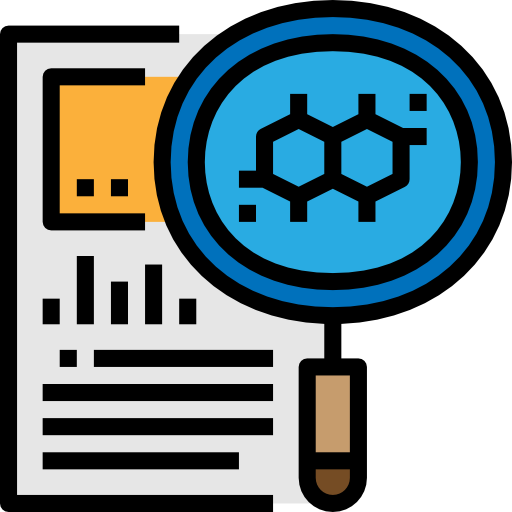
Kik vagyunk?
Kutatásokat végzünk olyan új eljárások kifejlesztése érdekében, melyek hatékonyan támogatják az orvosi tudományterület előrehaladását (kutatásban és klinikai gyakorlatban egyaránt).
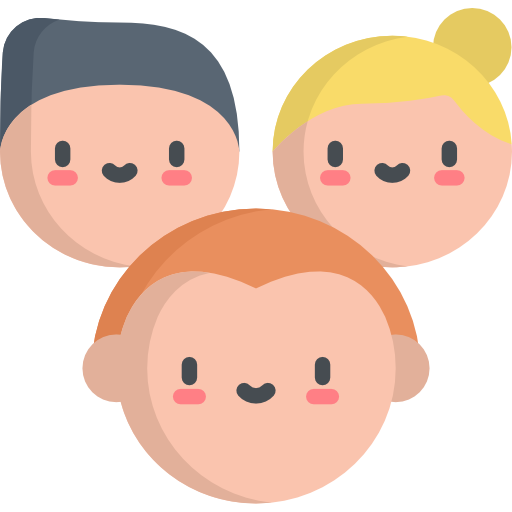
Csapatunk
Az élettani szabályozások központ kutatócsoportját a legszenvedélyesebb és legismertebb kutatók és tudósok alkotják az egészségügyi-mérnöki területen.
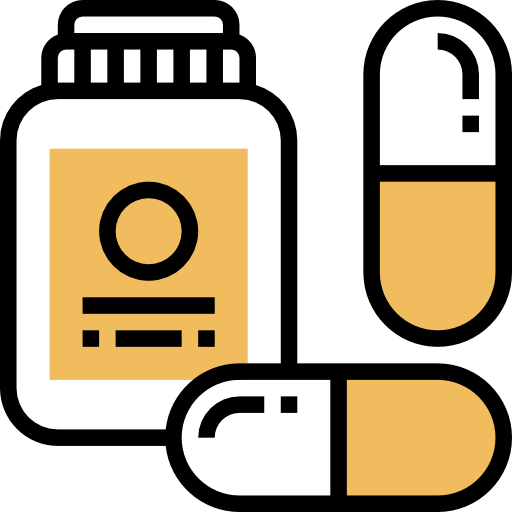
Daganatos betegségek
A Harvard Medical School bemutatta a tumor növekedésének angiogén gátlás alatt álló modelljét, amelyet tüdőrákos egereken végzett kísérletek segítségével validáltak.
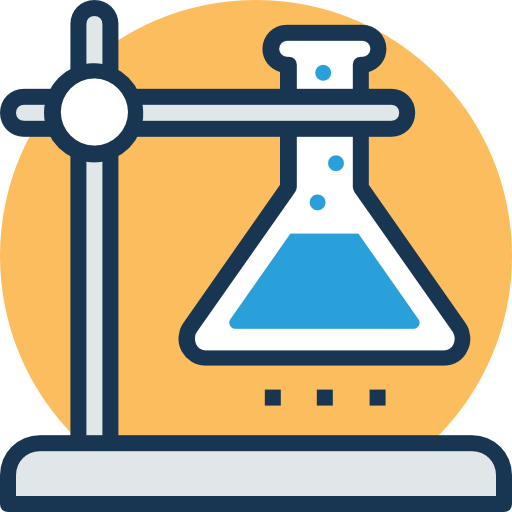
Új kutatás
Kutatási megbeszélést folytattak a PhysCon, a Harvard Orvosbiológiai Rendszertechnikai Kutatócsoportja, a Heim Pál Gyermekkórház és a Magyar Diabetes Szövetség között.